Machine Learning, Artificial Intelligence (AI) and Cyber-risk
The Evolution of Artificial Intelligence
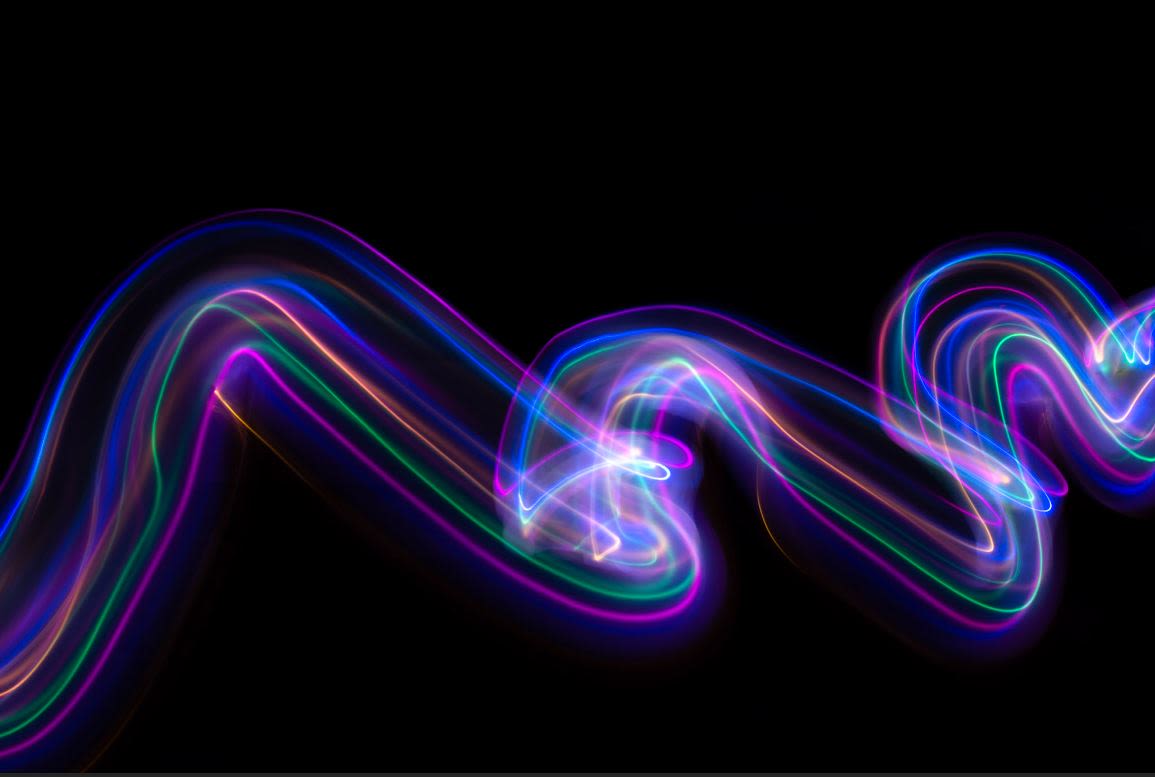
Artificial intelligence (AI) has been making headlines. We’ve seen the announcement of AI systems across a
range of industries tackling a range of different tasks.
Among other things, these systems have been able to successfully diagnose illness, drive cars, hold conversations, translate text, and create new images. But what is AI, and how has it evolved over the years?
For all the column inches that have been written about AI, a precise definition is hard to pin down. The term originates from a workshop held in Dartmouth in the summer of 1956. The original proposal said:
“An attempt will be made to find how to make machines use language, form abstractions and concepts, solve kinds of problems now reserved for humans, and improve themselves.”
Perhaps then the best definition of AI is programming computers to show “human- like” intelligence - whether that’s skill with
language, speech & images, or interpreting the world around us in other ways.
Over the years AI has encompassed a wide range of approaches. Early approaches to AI revolved around machine-readable
knowledge. Attempts were made to encode human understanding in a knowledge store and use search and logic to make inferences. For example, a system might know facts about the heights of buildings around the world. This information could be used with logical rules to answer questions like “which is taller, the
Empire State Building or the Eiffel Tower?”
However, human knowledge is messy, ever- changing, and full of contradictions and ambiguity. These systems could work for small tasks, but ran into too many problems when trying to store large amounts of knowledge. Knowledge bases are an essential part of some modern AI systems, but on their own are not enough to build truly human-level intelligence.
Over time, a more popular approach became to learn a model of the world from data. The current wave of AI is driven by this idea, and in particular by the success of a group of algorithms under the umbrella of ‘machine learning’ (ML). These algorithms don’t list out exactly the instructions that a computer should follow to complete a task, but they learn how to behave by being presented with a variety of data and uncovering the patterns in that data, a process dubbed ‘training’. A computer learning to transcribe audio would be trained on manually transcribed audio. A model learning how to identify topics in text would be trained on text data annotated with topic. These models could then be used to transcribe new and unseen audio, or categorise the topic of new text articles.
One particular family of model known as ‘deep neural networks’ has had big success in the past decade. Neural networks have been around for a while, but it’s only recently that there’s been enough data and computing power to effectively take advantage of their power. A modern speech recognition model for example
might look at hundreds of thousands of hours of audio data. A language model might be trained on billions of words of text. Training on such large amounts of data is only possible due to modern data storage and computing power. Deep neural networks have also been remarkably successful across different tasks - language, vision, audio - meaning that advances from one task can be rapidly applied to other tasks.
To get the large amounts of data needed for training the current generation of models, text and images are often scraped from the internet. But, data scraped from the web is not always representative of real life. A model trained on text collected before 2020 would know nothing of Covid-19 and all the changes it has brought to our lives. Language evolves all the time as new events take place. Keeping up-to-date is a challenge.
Web text also contains other biases. Wikipedia, for example, is an important source of text data for many machine learning models. Yet, biographies there skew towards Western figures and less than 20% are of women. This impacts the kinds of patterns that models learn. There’s an ever-growing list of machine learning models learning and reproducing stereotypes from the data they’re trained on. One example is sentiment analysis models associating
African American names more strongly with negative emotions than European names. Another commonly cited example is machine translation models which need to handle the translation of gender neutral language, such as in Turkish where the word ‘o’ means both ‘he’ and ‘she’. The models are likely to fall back on skewed patterns of language that are present in the training data, leading to translations such as “He is a doctor, she is a nurse”. Mitigating these kinds of biases is hard, and is a topic that’s currently receiving a lot of attention.
Despite these issues, machine learning is behind many of the AI products we use today - virtual assistants, web search, fraud detection, face ID, post recommendations on social media, and much more. It’s being used daily by large numbers of people around the world, and shows no sign of slowing down any time soon.
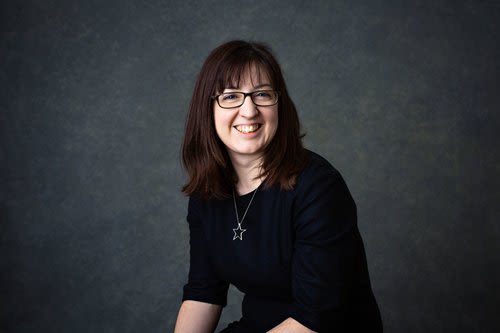
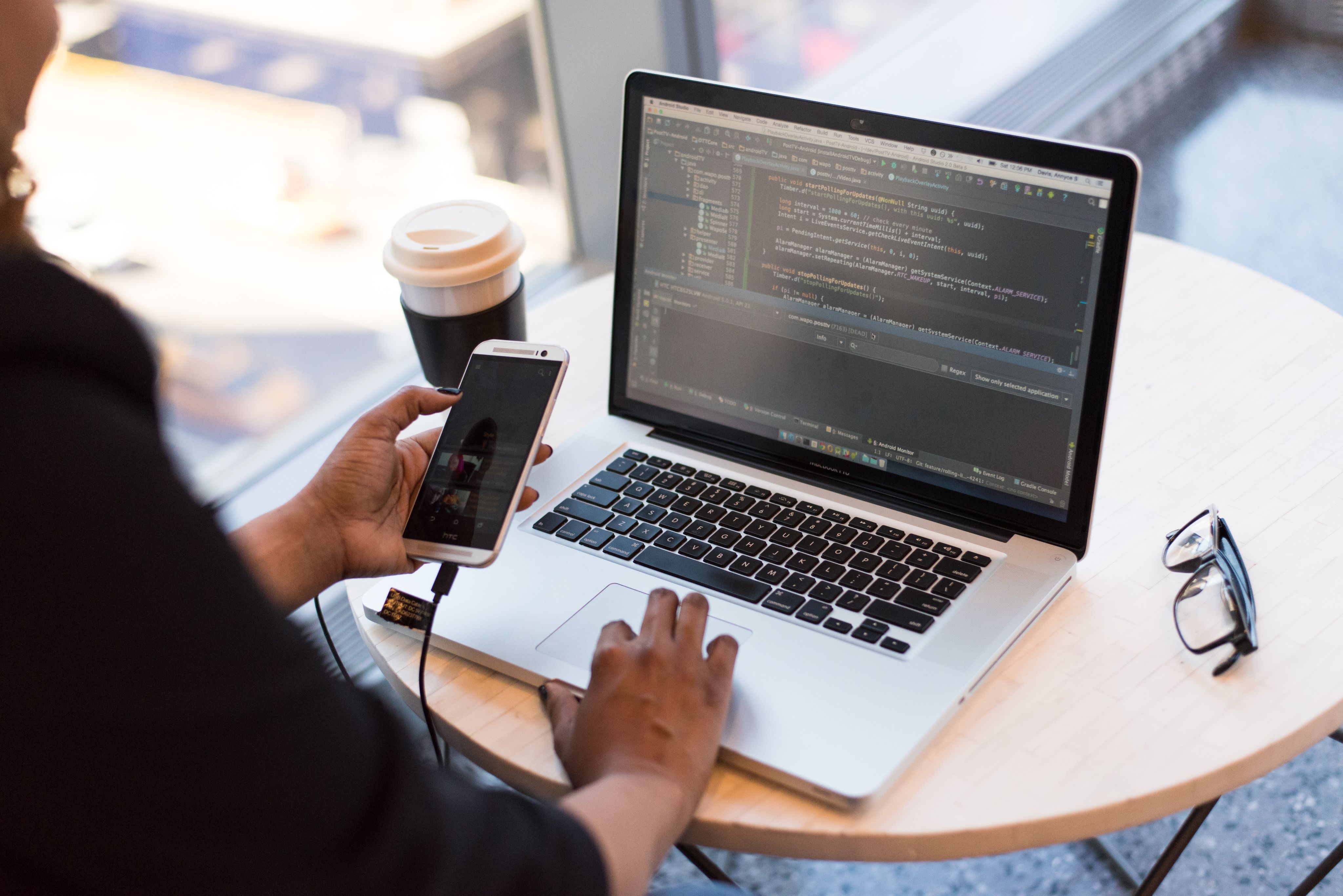
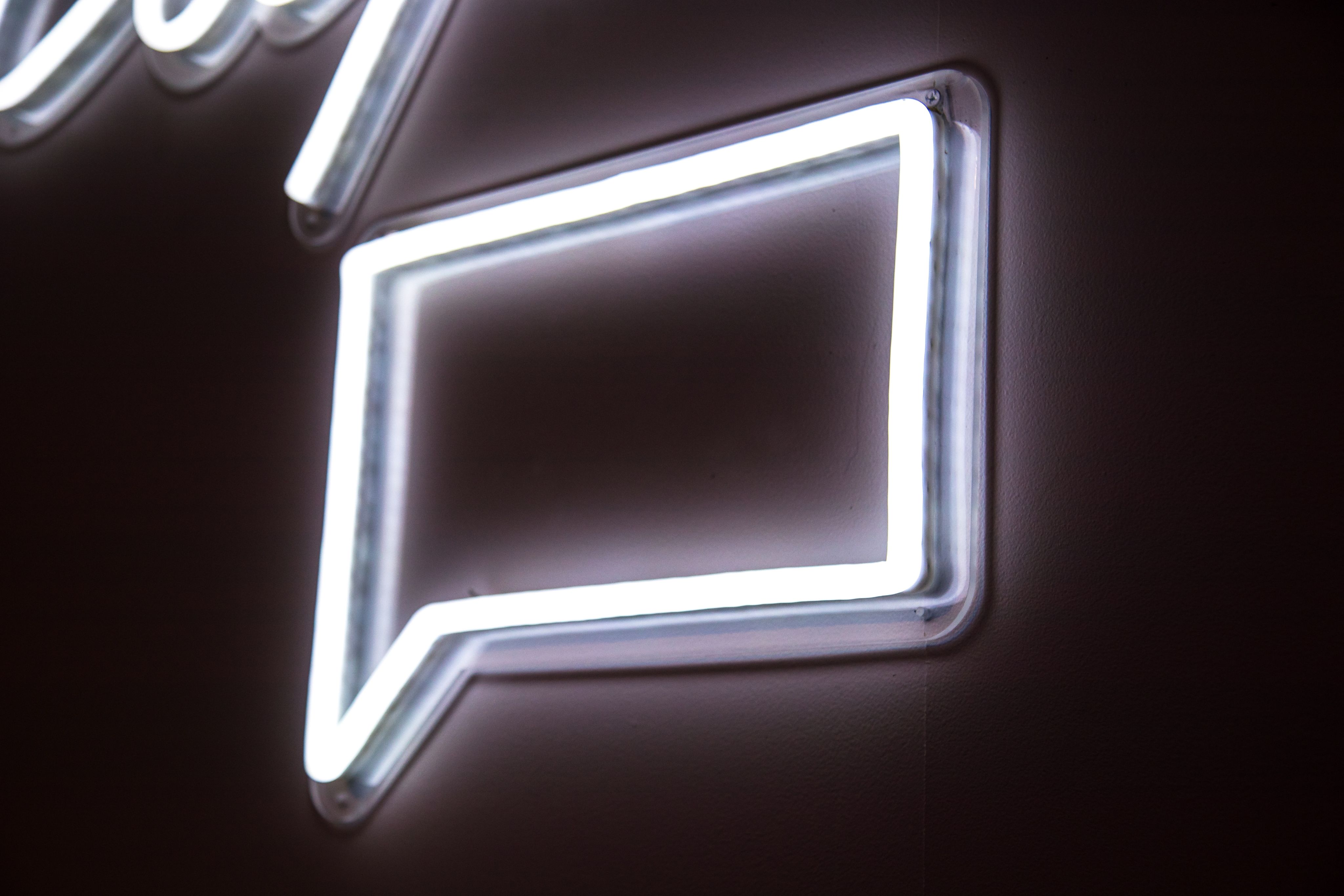
The Darwinian Summer 2022
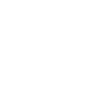